The Future of NLP in Data Science Applications
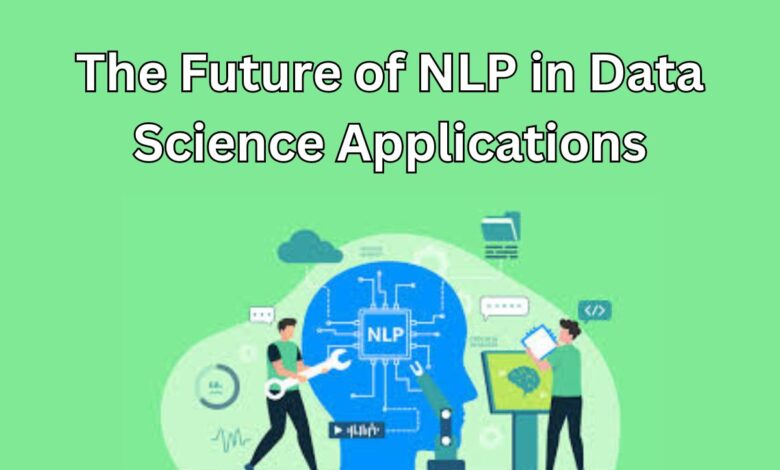
Introduction
Natural Language Processing (NLP) is one of the most exciting and rapidly evolving areas in data science. NLP focuses on enabling computers to understand, interpret, and generate human language in a way that is both meaningful and useful. It is the technology behind chatbots, voice assistants, language translation services, and much more. As data science continues to evolve, NLP is set to play an increasingly significant role in transforming the way we interact with technology and make sense of large volumes of unstructured text data. Let’s explore how NLP will shape the future of data science applications.
NLP in Data Science Applications
1. Current Use of NLP in Data Science
NLP has already made significant strides in various applications, some of which you may encounter daily:
- Chatbots and Virtual Assistants: Siri, Alexa, and Google Assistant use NLP to understand spoken commands and respond to queries.
- Sentiment Analysis: Companies use NLP to analyze customer reviews, social media posts, and feedback to gauge public opinion and sentiment.
- Machine Translation: NLP enables services like Google Translate to convert text from one language to another with increasing accuracy.
- Text Summarization: NLP techniques help condense long documents into brief, meaningful summaries, making information more accessible.
- Speech Recognition: Systems like Apple’s Siri and Amazon’s Alexa rely on NLP to accurately transcribe spoken language into text.
These examples only scratch the surface. As technology advances, NLP’s role in data science will expand, offering new capabilities and transforming industries.
2. Key Drivers for NLP Growth in Data Science
a) Big Data and Unstructured Text
The vast majority of data generated today is unstructured, much of it in the form of text—emails, social media posts, blogs, research papers, and more. Traditional data analysis tools struggle to extract meaning from this type of data. NLP can help unlock insights from unstructured text, enabling companies to analyze large amounts of written or spoken language effectively.
b) Advances in AI and Machine Learning
NLP relies on machine learning techniques to build models that understand and generate language. With the continuous improvement of AI algorithms, especially deep learning techniques like transformers (e.g., GPT-4, BERT), NLP models are becoming more accurate and capable. These models can now understand context, detect nuances in language, and even generate human-like text.
c) Improved Computing Power
One of the reasons NLP is advancing so rapidly is due to the increased computational power available today. Complex NLP models require significant resources to process large datasets and perform tasks such as translation or speech recognition in real-time. With the advent of cloud computing and powerful GPUs, running these resource-intensive models has become more feasible.
d) Multilingual Capabilities
The ability to process multiple languages is becoming a crucial feature of NLP. In a globalized world, businesses need tools that can interpret and generate text in various languages. NLP models are increasingly trained on multilingual datasets, breaking language barriers and enhancing communication.
3. Emerging Trends in NLP
a) Conversational AI
The future of NLP will likely see more sophisticated conversational AI systems that can engage in natural, human-like conversations. These systems will be able to handle context-switching, understand ambiguous queries, and respond with nuanced answers. This can enhance customer support, healthcare, and education services.
b) Low-Code NLP Platforms
As demand for NLP applications grows, low-code or no-code platforms that allow users with minimal programming knowledge to build NLP applications will become more common. These platforms will democratize access to NLP technology, enabling businesses to leverage its power without needing highly specialized teams of data scientists.
c) Personalized NLP
Future NLP models will likely become more personalized, tailoring their outputs based on user behavior, preferences, and interactions. For example, virtual assistants will be able to understand individual user preferences, remember past interactions, and offer more relevant responses over time.
d) Ethical NLP
As NLP becomes more integral to decision-making processes, the need for ethical considerations becomes paramount. Future developments will likely focus on creating models that are free from bias, promote fairness, and respect user privacy. This trend will be essential as NLP is increasingly used in sensitive areas like healthcare, hiring, and law enforcement.
4. Applications of NLP in Data Science
a) Healthcare
In healthcare, NLP can analyze electronic health records (EHRs) to extract critical information about patient history, symptoms, and treatment outcomes. By automating the extraction of insights from medical documents, NLP can assist doctors in diagnosing conditions faster, ensuring better patient care.
b) Finance
NLP is becoming a powerful tool in the financial sector, where it can be used for tasks such as analyzing news articles, market reports, and social media posts to predict market trends. Sentiment analysis helps investors gauge public reactions to events and make informed decisions.
c) Customer Service
NLP enhances customer service by powering chatbots that understand natural language and respond intelligently. It can automate routine inquiries, providing instant responses to customers and freeing up human agents to handle more complex issues.
d) Education
In education, NLP can be used to build intelligent tutoring systems that assess student responses and provide feedback in natural language. It can also help in grading assignments, detecting plagiarism, and summarizing large volumes of academic text.
e) Marketing
In marketing, NLP can analyze customer feedback, reviews, and social media posts to understand consumer preferences and sentiments. This enables businesses to tailor their marketing strategies and improve customer satisfaction.
5. Challenges in NLP
Despite its progress, NLP still faces several challenges:
- Ambiguity in Language: Human language is inherently ambiguous. Words can have multiple meanings, and the same sentence can be interpreted in different ways based on context. This makes it difficult for NLP models to achieve 100% accuracy.
- Language Diversity: Developing models that can handle multiple languages or dialects accurately remains a challenge. Many NLP models perform well in languages like English but struggle with less widely spoken languages.
- Bias in Data: NLP models are only as good as the data they are trained on. If the training data contains biases, the models will reflect those biases. This can lead to unfair outcomes, especially in areas like hiring or law enforcement.
- Resource-Intensive: Developing and running large NLP models requires significant computational power, making it costly for small organizations to implement.
6. The Road Ahead
As we move forward, NLP will continue to be a game-changer in data science applications. Its ability to process and understand natural language will enable businesses to extract more value from their data, enhance customer experiences, and make more informed decisions.
With advances in AI, machine learning, and computing power, the possibilities for NLP are virtually limitless. From improving healthcare outcomes to powering intelligent customer service, the future of NLP in data science holds immense potential. However, ethical considerations, challenges related to language diversity, and the need for robust, unbiased models must be addressed to ensure NLP’s positive impact on society.
Conclusion
The future of NLP in data science is bright. As technology evolves, we will see even more powerful and sophisticated applications of NLP that will transform industries, improve services, and create new opportunities for innovation. However, careful attention to ethical issues and challenges will be crucial in ensuring that NLP benefits everyone. The Best Data Science Course in Noida, Delhi, Gurgaon, and other locations in India can play a pivotal role in helping individuals and businesses prepare for the exciting advancements in NLP and data science.